Mechanical Reproduction in the Age of Algorithmic Generation
By Alex Kauffmann
Illustrated by Chengtao Yi
A reflection on Walter Benjamin’s 1935 influential article on art, like photography, that could be easily reproduced. But at least they started with something real. In the age of algorithms, that’s not a certainty.
“Even the most perfect reproduction of a work of art is lacking in one element: its presence in time and space, its unique existence at the place where it happens to be.” (1)
Walter Benjamin’s 1935 essay “The Work of Art in the Age of Mechanical Reproduction” is mandatory reading for first-year students of art, film, and cultural history for a very good reason. The essential questions it raises for art have resonated with a new timbre for each generation. Among the essay’s principal conceits is that mechanical reproduction (photography, film) erases both the dings and stains and burnishing that time and circumstance bestow on a physical work of art, what he calls its “aura,” and all references to the means of its own production. Brush strokes on canvas give a painting away as such, but great pains are taken when making a film or taking a picture to ensure that no sign remains of the booms, mics, Klieg lights, tripods, and people required to produce it. A new kind of ahistorical reality is produced by mechanical means, one that Benjamin in his essay worries is by necessity a tool of politics. We’re hearing the echoes of that same concern in our own time, when new digital technologies can generate photorealistic images entirely algorithmically — no need for mechanical capture or reproduction.
“The sight of immediate reality has become an orchid in the land of technology.”
In 2009, a video editor and animator named Duncan Robson cut together “Let’s Enhance”(2), a montage of scenes from TV shows and movies in which computer techs “enhance” blurry surveillance images, uncovering crucial clues in the newly resolved detail. After he posted it on YouTube, it quickly made the rounds among digital creatives, ad folks, and film people who all knew perfectly well that upscaling a digital image only enlarges its existing pixels; there’s nothing lurking between them. “Let’s Enhance” was the creative class collectively smirking at a credulous public that believed a photograph captured a moment in its entirety, and that, given the right filters, it might reveal some previously imperceptible aspect of reality.
Ten years later, the joke isn’t as obvious. Automated techniques for high-dimensional statistical modeling — a less sexy but more descriptive moniker for what is commonly referred to as “machine learning” or “AI” — have made it possible to generate photorealistic imagery ex nihilo(3). Most of the resulting synthetic imagery is still relatively clunky and easily recognized, but progress is swift, and in certain limited image domains (human faces, for instance), detecting algorithmic generation requires significant scrutiny. Synthetic images produced by algorithmic techniques have become sufficiently difficult to differentiate from those captured by a camera that certain academics, congresspeople, and journalists have begun to, for lack of a better term, freak the fuck out.
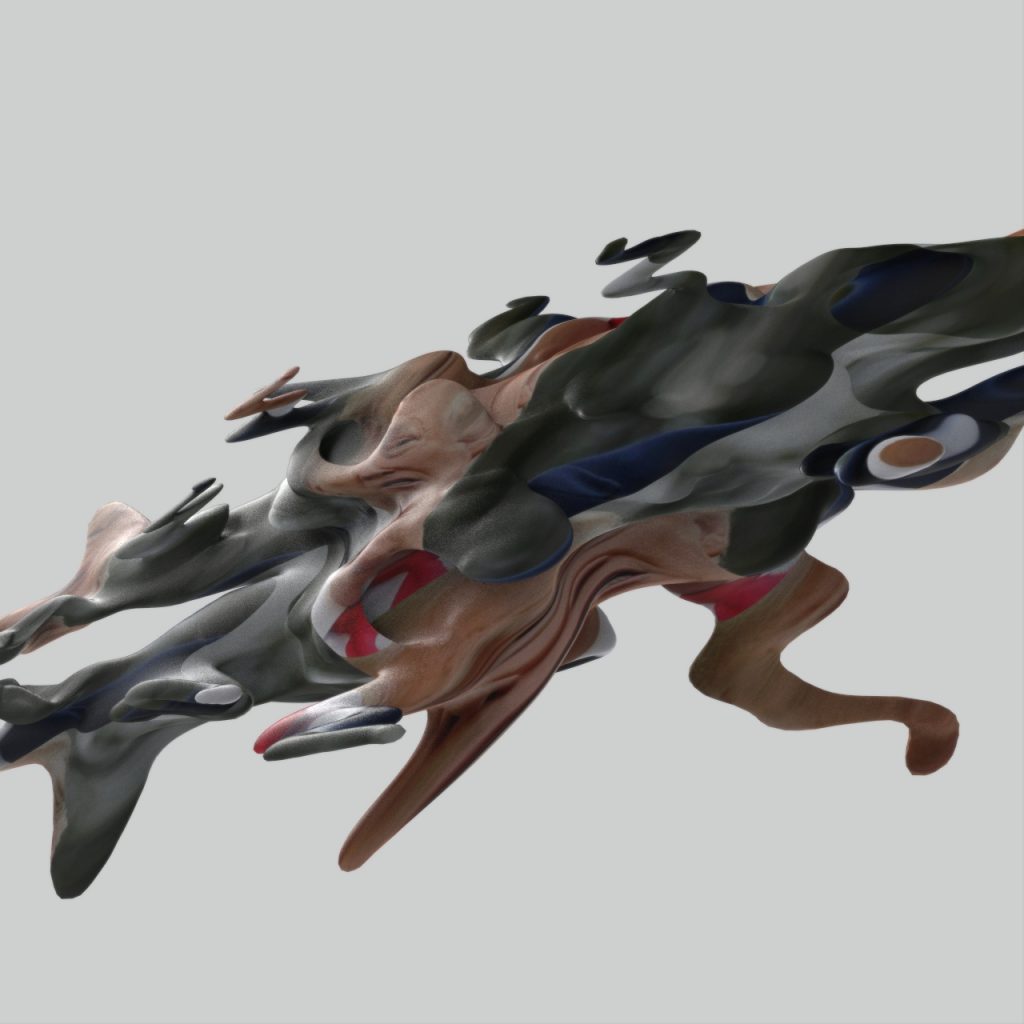
“The authenticity of a thing is the essence of all that is transmissible from its beginning, ranging from its substantive duration to its testimony to the history which it has experienced. Since the historical testimony rests on the authenticity, the former, too, is jeopardized by reproduction when substantive duration ceases to matter. And what is really jeopardized when the historical testimony is affected is the authority of the object.”
Danielle Citron, a law professor at the University of Maryland who studies harassment and hate crimes online, has written extensively on the danger of algorithmically generated fakes in which she articulates the alarmist point. She argues that when anyone can easily produce false images — these being worth a thousand words and thus “stickier” than written falsehoods — untruth will spread proportionally faster across social media, causing irreversible reputational and psychological harm to good people, allowing bad people whose misdeeds are recorded to hide behind claims of fakery, and ultimately undermining trust in authority, institutions, and the very fabric of civil society.
Ironically, the dangers she warns of arrived some time ago, without algorithmically generated images. There are daily news stories of online reputational and psychological harm. The crying of fake news and the undermining of institutions have defined the global media landscape since the last presidential election or before. Facebook and Twitter have long been rife with bullshit that spreads across the earth like a brush fire, so why all the fuss about images in particular?
The canary in this particular coal mine is a flavor of face-swapping algorithm that maps a face from a source video onto another face in a destination video to produce a “deepfake.” The algorithm has mostly been used to put celebrities’ faces onto pornstars and to make Nick Cage star in movies he was never in. There have been occasional attempts to use deepfakes in a political context, like this video, a Belgian political party posted on Facebook showing Donald Trump belittling Belgium’s environmental record. The wonky mouth makes it easy to recognize as a fake, but the specter of world leaders saying whatever anyone wants them to is very real to synthetic imagery alarmists.
A lack of understanding of the underlying technology is partly to blame. Over the last five years, academic computational imaging conference papers have shifted from using images of 3D teapots and fluorescent-lit graduate students to images and videos of public figures. When a research group in Germany published Face2Face, a technique that maps one face’s motion onto another, they demonstrated it by having graduate students puppeteer videos of world leaders. A year later, researchers at the University of Washington presented a refinement that better synched mouth movements in a video to an arbitrary audio track using a video of Obama. Someone who doesn’t read the papers or understand machine-learning algorithms might be forgiven for seeing the end of days in academia’s apparent zeal to embrace the most nefarious applications of their work.
The truth is much less sinister. Because machine learning algorithms demand huge quantities of source data, computer scientists almost by necessity rely on video and images of public figures to ensure they have sufficient training data to produce good results. As the University of Washington researchers above note in their lip synching paper:
“Barack Obama is ideally suited as an initial test subject for a number of reasons. First, there exists an abundance of video footage from his weekly presidential addresses—17 hours, and nearly two million frames, spanning a period of eight years. Importantly, the video is online and public domain, and hence well suited for academic research and publication. Furthermore, the quality is high (HD), with the face region occupying a relatively large part of the frame. And, while lighting and composition varies a bit from week to week, and his head pose changes significantly, the shots are relatively controlled with the subject in the center and facing the camera. Finally, Obama’s persona in this footage is consistent—it is the President addressing the nation directly, and adopting a serious and direct tone.”
The researchers allude to another reason why the fears of synthetic imagery are overblown. Getting even a mildly good result requires tons of data. The videos in academic papers are cherry-picked best cases. Anyone actually trying to train their own models quickly runs into the limitations they allude to above: the need for large amounts of consistently lit, high-resolution, not-too-dynamic close-up video of the subject. Citron might argue that while that’s currently true, the underlying models may someday soon get so good that they’ll achieve believable results with only a single video of any person. But that’s largely beside the point, because believable synthetic imagery is already ubiquitous.
Instagram and Snapchat and all their East Asian doppelgangers have normalized idealized and synthesized images. People’s sophistication tends to keep up with technical advancements, as it always has. But it requires work; it requires us to disbelieve what we see. Jordan Peele used a video of Obama to warn about the dangers of these techniques in a recent PSA. He asks people to question where their information comes from on the Internet, but does not inveigh against the format because algorithmic image enhancement is at this point inescapable.
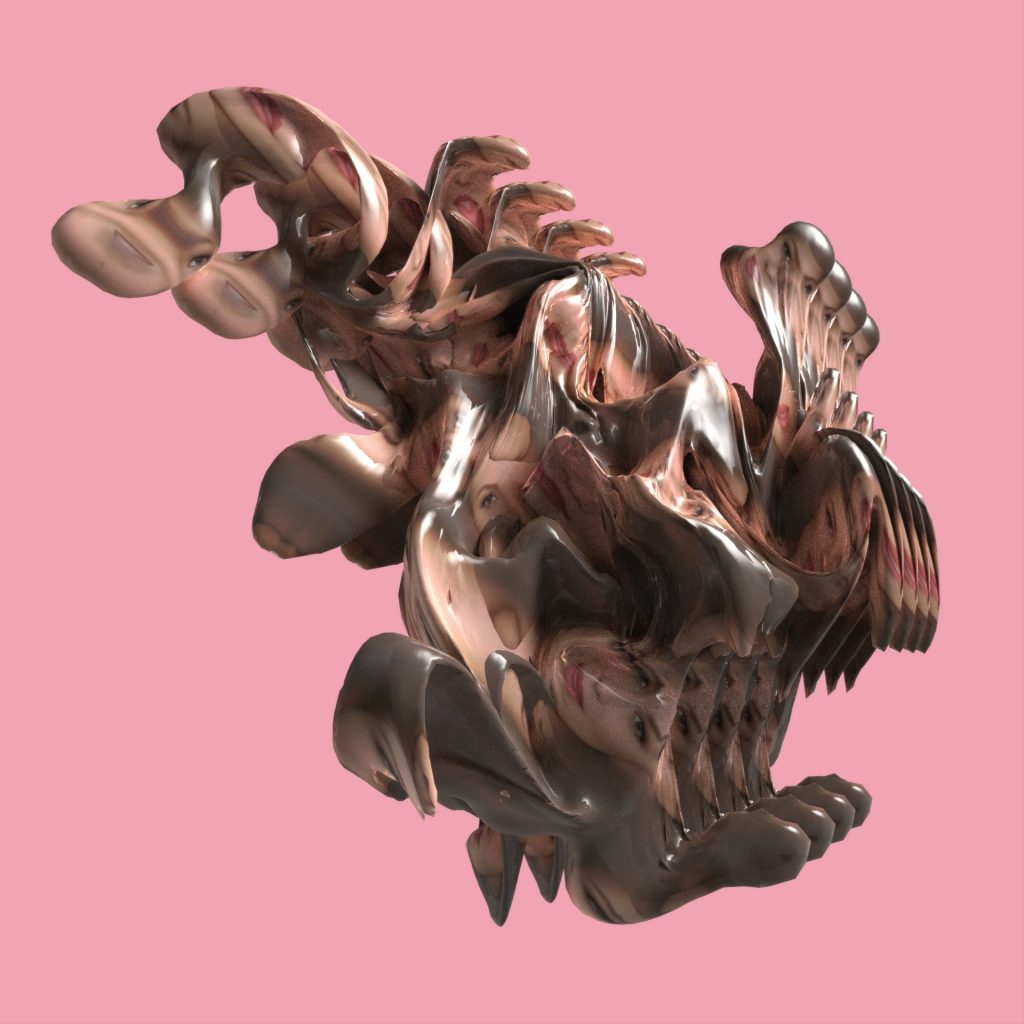
Technically, every image captured with a digital camera has already undergone numerous algorithmic transformations and processing steps to render it to the screen. Many times, the images themselves are amalgams, products of techniques such as HDR and Google’s recent Night Sight, which merge a burst of individual images into a single image to achieve a dynamic range that more closely resembles what our eyes perceive. This point may seem pedantic, but it does raise the question of where to draw the line between “real” and “synthetic.” Photojournalists have reached an uneasy detente that permits whole-image processing for aesthetic reasons but decries adding or removing pixels, which risks manipulating the interpretation of the image. But even that line is blurry, since it’s generally acceptable to remove dust or other image sensor artifacts from an image, for instance.
A current deeper than technical ignorance stirs the fears of synthetic imagery. There’s an underlying political narrative surrounding the means of production that Benjamin would definitely recognize. It’s been possible to alter a photographic image since the advent of photography. But, the argument goes, until now, only governments or Hollywood studios could afford the time, talent, and resources to alter an image convincingly. With the new generation of digital tools, anyone will be able to create any image and disseminate it globally in an instant. Is that actually more dangerous than a corporation or government doing the same thing? And, as we’re seeing right now, you don’t need images to spread falsehoods.
Technology is governed by social agreement, sometimes more successfully than others. Journalists don’t Photoshop their images, and when they do, they get in trouble. Scientists don’t invent results, and when they do, they get in trouble. But what happens when regular people “make” photorealistic images that don’t accurately portray something that actually happened on their phones? We’re standing at the fault line between two paradigms, where ideas of photography as faithful evidence of reality clash with a new conception of reality itself as a medium. Digital tools and techniques have unlocked the potential of photorealism as a material like paint or ink. They effectively capture realities that are un-mechanically reproducible, re-embedding pictures within the fabric of all of the images that came before and opening up brave new artistic possibilities. To those who worry that reality is in danger, I say: let’s enhance.
1. All quotations, unless otherwise noted, are from Walter Benjamin’s essay.
2. This video itself has been enhanced! Robson remastered his original 2009 supercut with higher resolution footage and better quality audio for the Cut Up exhibition at the Museum of the Moving Image in 2013.
3. That’s not exactly true. These images don’t actually come from nothing. Rather, they’re collections of pixels statistically determined to produce photorealistic images, the result of probability distributions learned from billions of actual photographs.
Alex Kauffmann (ITP 2010) is Technical Project Lead at Google’s Advanced Technology and Projects Group. He led the design of Google Cardboard.