By Michelle Shevin
Illustrated by Chengtao Yi
Is technology good or bad for the future? Should we be excited or scared of new technological developments? Perhaps we're asking the wrong questions. This is not an essay about technology, but an essay about systemic inequality.

In his 2005 book, What Do Pictures Want?, WJT Mitchell wrote that our time is “best described as a limbo of continually deferred expectations and anxieties. Everything is about to happen, or perhaps it has already happened without our noticing it.”
Nearly 15 years later, we’re in the midst of assembling a surveillance architecture designed to ensure that even the minutiae of our lives won’t be beyond notice. Satellites that can detect the books on your coffee table. Doorbells that double as neighborhood nanny-cams for the police. Face-recognizing CCTV with an authoritarian back-door. Wearables counting our steps and modulating our insurance premiums. Real-time location from our mobile phones sold to bounty hunters. Speakers recording our most intimate and banal moments for machine — er, human translation.
This militaristic vision of safety, in the form of “total information awareness”, commits to policing a symptom (human behavior) while underwriting the deeper disease: a narrative of dominance, consumption, and growth that directs our institutions, shapes our conceptions of progress, and threatens our future on this planet.
This is not an essay about technology, so much as it is about entropy.
The Future is a Business
For decades we have had an industry, though relatively few realize it exists. It’s a sort of discipline of practice, devoted to tracking and unpacking the present, all under the guise of predicting what happens next. Deployed in the service of operational goals, the project here is not just to anticipate possible futures, but to actively create the future.
This is the futurology industry (whose practitioners are often called “futurists,” not to be confused with practitioners of the 1920s Italian art movement of the same name). Futurology is a deeply flawed, sometimes ridiculous, and for many years, closely guarded set of tools for collaborative sensemaking (“trend sensing,” “forecasting,” “horizon scanning”) and strategic planning (“opportunity discovery,” “scenario planning,” “strategic roadmapping”).
Since the industry’s rise in the mid-20th Century, these world-building tools have typically been operated by institutions of power as closed processes in contexts dangerously supportive of —even reliant on—the status quo.
As a distributed project largely only accessible to those in positions of power and privilege, the central, if not explicit, goal here is to enact one of many possible futures. And to construct, measure, and police conceptual and structural borders and boundaries that ensure the general continuity of business-as-usual.
For the multinational corporations and government agencies with the budget to ingest its $50,000 syndicated research subscriptions, bespoke opportunity discovery workshops, and customized consulting contracts, the futures industry promises early warning on the future. But signposts on the road are not enough. Futurists also sell the strategic foresight to land at the top of any number of possible societal configurations, and the lead time to devise machinations to make them more or less likely to occur. This is full-stack futuring: it is much easier to read the tea leaves if you have been genetically engineering and then trimming them yourself.
Much of this business of tracking and prognosticating appears to center on technology. This focus on the shiny, new, and networked, is not only due to a close industrial allegiance to Silicon Valley (although it is futurology’s intellectual and historic home in the United States), but also because as a window into shifting power relations, technology change provides a way to explore the strength and resilience of deep structures: laws, regulations, narratives, and planning processes.
The Bureaucratic Imaginary

For decades, dedicated futurists have tracked progress on distributed sensors, batteries, computing speed, processing power, spectrum availability, data volume and veracity, machine learning, and other enabling technologies that promise to embed computational forecasting as a layer of infrastructure across private and public systems, often under the heading of “cyber-physical systems”—long before the “revolution” was heralded and called “big data,” “AI,” or “automation.”
And as predicted, this software revolution first “ate” the private sector and is now rapidly colonizing the public sector, being implemented at every level of government around the world.
Planning for the future is much simpler if we assume linearity and knowability through quantitative data. However, through the logic of machine learning, we’re conflating pattern-identification and sensemaking.
The automation-via-analytics fantasy has given up on human judgment. It implicitly prefers discrimination-at-scale and by-design over the unpredictable dice throw of decentralized human prejudice (and institutional programming). But this preference also extends over human capacity for nuanced reasoning and contextualized decision-making. It tinkers, optimizing at the margins, incrementally nudging toward “improved outcomes” (only ever the ones that are measurable). It bars even the possibility of fundamental reorientation based on revised first principles.
What’s more, we need to acknowledge how those marketing fantasies and policy scenarios have limited our imaginations…Billions of dollars later, we’re still pursuing the same old dreams.
– Shannon Mattern
As we predict, we plan. And we plan to measure the success of our plans according to the outcomes we plan to achieve.
Most of our metrics are designed to track the trajectory of individuals under conditions of systemic machinations. A process change here, an updated standard there, a technology stack, a new policy, a “disruptive innovation!” To know the effects of any implemented plan, we measure the behavior of individuals, the essential unit of control.
Alas, facts don’t change minds. But data can tell stories.
Forecasting, planning, measurement, and evaluation operate as an ouroboros: a feedback loop that relies on and produces mounting amounts of individual data, as a sort of toxic exhaust. Importantly, this data, whose very design and collection have been shaped by a particular set of values (often: neoliberal efficiency), is framed as objective “evidence” and the best (or only) source of informed decision making. Manipulated through computational recipes called algorithms, data can train systems to both predict and automate the outlines of the future. We must now recognize, in Ruha Benjamin’s words, “the weathermen who make it rain.”
This mathematical decoupling of data from the context of the structures, systems, stories, theories, and values that drove its collection and shaped its borders—under the guise of predicting the future— is “statistical malpractice”, smoke and mirrors. We’re being captured, blindfolded, and taken for a ride by our own deafening meta-narratives of who we are and what we think we deserve.
Our future is eating itself. It dreams of transcending its body. It dreams of first nuking, and then rocketing to and colonizing Mars, leaving its home, the planet, a dried husk or shed cocoon. Our future sees itself as separate from that home. It not only accepts these dreams, it treats them as axioms. It cannot imagine anything else. It plans on them.
A Cyborg Dream of Electric Inequality
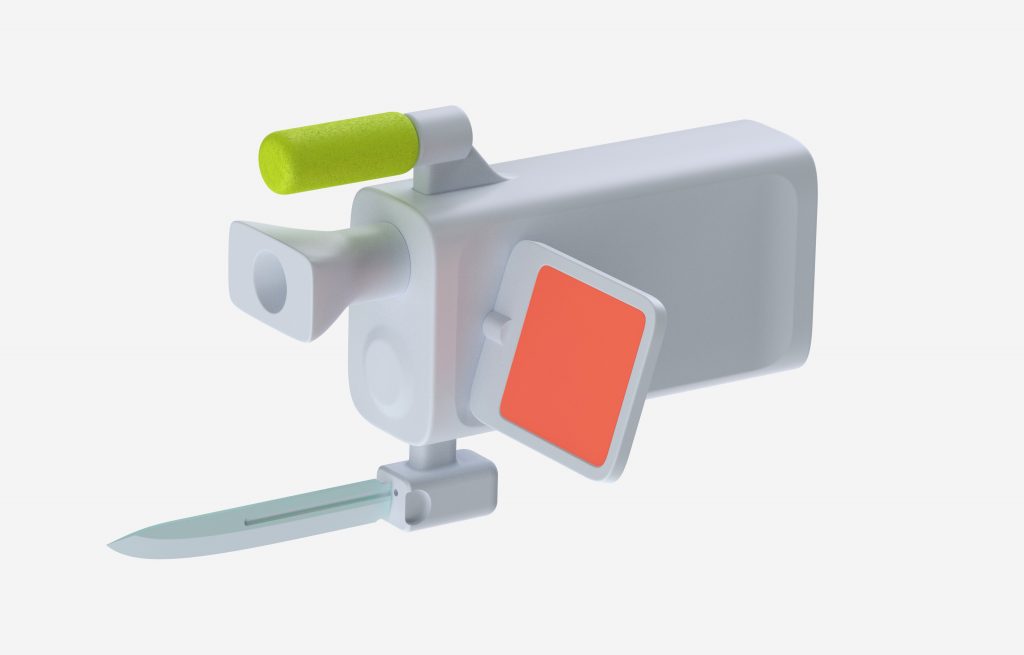
There are many elephants in the room of futures thinking, but none as pressing as the future of forecasting itself. Futurists have long relied on the business of public sector planners. But the logic of targeted advertising has arrived: everything is measurable, knowable, predictable, and plannable, through data.
Legal restrictions intended to limit surveillance architectures, by preventing large scale data sharing, are largely surmountable and come with their own externalities. Incentivizing the inclusion of law enforcement data to make use of “public safety” exceptions, is one example. Many of the worst current examples of automation-via-data-integration sit at the intersection of educating children and calling the police.
In this technocratic dreamscape, civil servants have been freed from the drudgery and ambiguous work of making important determinations about individuals and their interactions with public systems. Should she be denied bail? Might that child someday be expelled or arrested? Does he really need a home health aide? Should we take away their daughter?
This project is fundamentally about telling machines about individuals in order to train those machines to manage people. Over time, so the fantasy goes, requiring less and less human oversight.
The trick, of course, is that the line between human and machine is one of those boundaries that blur as you look closer. The training data collected through human logics about humans, must be reviewed, cleaned and labeled by humans (the same reason people are listening to our incantations to Alexa), its relevance for inclusion in integration efforts determined by humans, the algorithms that combine and sort it designed by humans, targets of optimization chosen by humans, informed by human values, defined through human institutions, structured by human power relationships…
Machining Morality
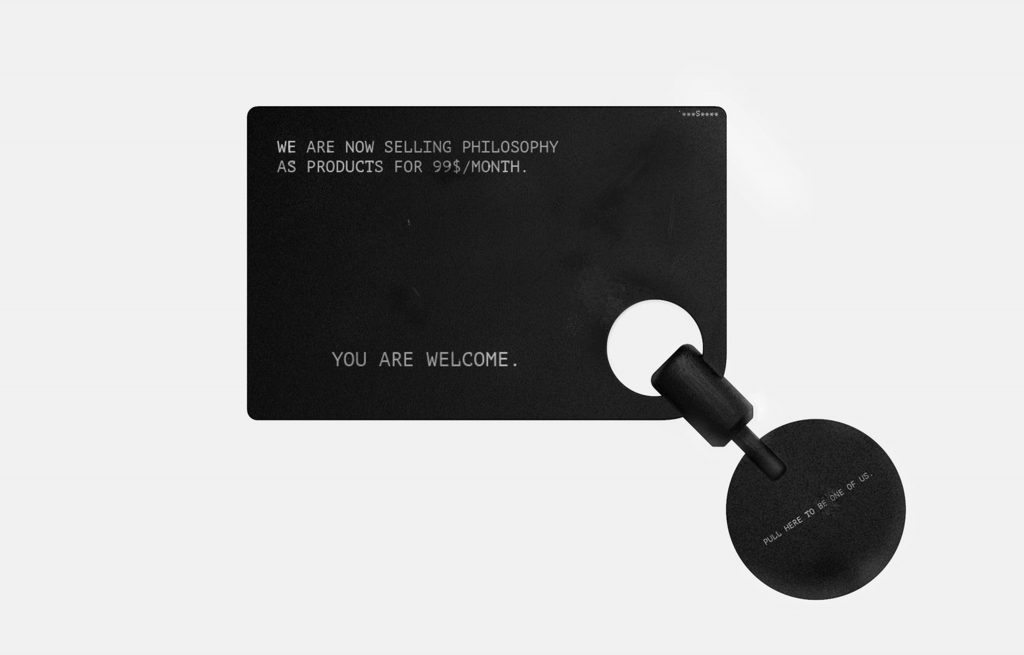
In marked contrast to the marketing hype around the “power of AI to change the world,” data scientists themselves have long been alarmed by what data is being asked to do. Disciplines from computer science to anthropology, STS, and design, are generating useful critiques and interventions.
But some of these may reinforce a problematic narrative of inevitable technological progress. Investing in the fairness, accuracy, and transparency of the algorithms, while important, also suggests that eventually, the machine may make itself readable to, redressable by, and responsible for humanity.
While critically important to generate, critiques of “bias” in AI may imply that a technical fix is possible; that the fault exists as something to be measured and corrected for as a sort of mathematical adjustment. Algorithms can be “audited” for fairness. Code can be tweaked to be “race-blind.”
Likewise, “solving” for privacy via technology ignores (and may propel) personal and systemic harms that are only hinted at by the concept of privacy. Exposure. Exploitation. Vulnerability. Loss of control of our own narrative. Being haunted by the worst thing that’s ever happened to us. A buggy misunderstanding that lingers like a bad smell.
Even entirely reasonable arguments positing that technologies such as face recognition, are not “ready for primetime” because they have not been trained inclusively on diverse populations and therefore fail to recognize non-white faces, likewise fail to miss the point.
Inequality is a feature of capitalist institutions, not a bug.
And the machine is us. The useful distinction here is not in some fundamental separation between man and machine conferring objectivity and neutrality upon the latter, but in the appearance of difference that permits a recapitulation and reification of capitalist institutional power, under the guise of technological progress.
Automated social service delivery systems are fundamentally about, as Virginia Eubanks puts it, “managing the individual poor in order to escape our collective responsibility to eradicate poverty.”
Before we outsource planning for the future to computational analytics,
Can they ever be a tool of systemic transformation?
Can they see systems, or are they blinded by our myopic measurement of individual outcomes?
Can they be directed by, and open to continual feedback from, impacted communities?
These are questions to contend with before embedding predictive analytics as a layer in planning and service delivery, not after the infrastructure is too big to fail.
Can they do moral work?
The work of improving communities is moral work. Not moral in any religious sense of the word, but in the sense that we must prioritize a different set of logics. This kind of work may require foregoing the default optimization targets of efficiency and cost savings, and instead take a deeper and harder look at the systems and structures that continue to generate inequitable outcomes, replicating the worst injustices of our past.
Can they imagine different stories?
Stories, Not Solutions
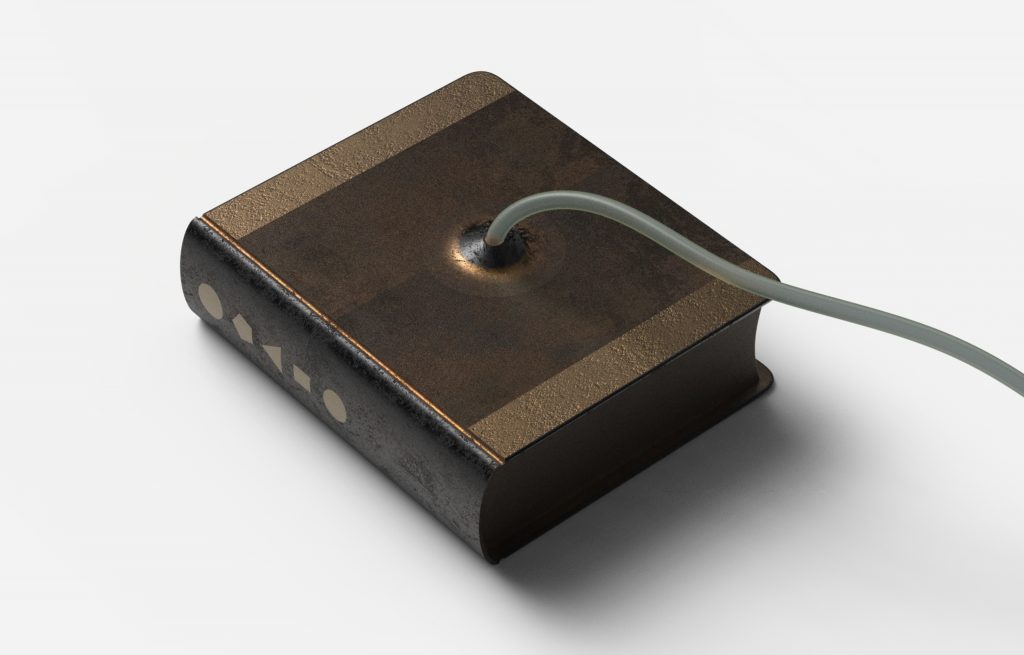
What would it look like to embed hope or aspiration into our vision of technological progress?
As long as we are stuck in myths about dominating “nature”, about othering our environment and each other, neither human nor machine logics will be able to transcend our ecocidal operating system.
As my colleague Salome Asega has pointed out, “the fear of superintelligent machines is really channeling something much deeper — a guilt and fear of machines doing unto humans what humans have done unto each other.”
We are something that the Earth is doing
– unknown
Can you start a real revolution without chaos? Maybe not. But if you could, it would start with a new story. Creating a new story starts with recognizing how fundamental storytelling is to the production of “evidence,” which our current planning and innovation infrastructure only absorbs through institutional touchpoints that confer “authoritative” analysis (read: elite institutions, large-scale data-sets, randomized (if not reproducible) controlled trials). As we have seen with MIT’s “future factory,” such institutions undermine the lived experience of the communities most impacted by public planning at a structural level.
It also means recognizing that our civic infrastructure was built for the normative perpetuation of a status quo that is morally wrong and fundamentally unsustainable. As a society, we don’t need to agree on policy choice, problem framing, or ideology. But can we agree to build the infrastructure needed to have those conversations? Can we move toward centering different stories?
This infrastructure won’t be delivered algorithmically. In lieu of infrastructure and apparatus for societal civic sensemaking, we’ve seen fit — tautologically absent any capacity to debate the trade-offs in clear terms — to automate decision-making via pattern identification and replication. We currently have a massive swath of our energy and resources invested in programming our future based on the flawed patterns of our past.
Algorithms, when commonly applied toward the optimization targets of efficiency or cost savings, have the effect of not only casting historical biases inherent in the data they are trained on into our plans for the future, but also function as a mechanical stifling of the space for imagining what true systems reform might look like. If we wish to use innovative tools in our pursuit of making communities better, that innovation must be in how we engage those most impacted by the injustice of our current systems.
Make no mistake: this isn’t a technology issue, but a civil rights issue. Unfortunately, there’s a lot of jargon (technical terminology, marketing hype, legalese), keeping communities from engaging in the wave of data collection and technology procurement that will shape how social services like education, welfare, and child protection are delivered for decades to come. There’s little space for the meaningful and collaborative radical reimagining of alternative futures when the public is repeatedly told that the revolution is already here and they just don’t understand it.
A Machine Readable Reality

Under the heading of solving problems, we are in the midst of a gold-rush to fit reality to machine readability.
Whether it’s “building a city from the internet up,” as Google sibling Sidewalk Labs has proposed to do in Toronto, or a retrofitting of sidewalks themselves for an autonomous car future, into “basically cages with defined doors that open only when the traffic lights are green so the world becomes simple enough for cars to ‘understand.’” This is the default imaginary data-driven future, made manifest.
Alongside the now ubiquitous “dark patterns” designed to extract ever more data from our physical and digital transactions and interactions, are the more subtle, long-term effects of algorithmic intermediation.
Ali Alkhatib puts it like this, “it’s not necessarily calamitous when we build systems that help us interpret the world in simplified ways, but it becomes a problem when those systems begin to reshape the world according to the simple, digestible, but hollowed out metrics that we developed initially to interpret the world.”
Just as we scarcely understand the bodily effects of a lifetime of exposure to chemicals in consumer products, the cross-sectoral ideological drive toward data collection will have long term impacts, many of them unintended. In particular, the resultant pervasive distribution of surveillance architecture throughout our built environment, just as we are pushing the limits of natural resource consumption and feeling the impacts of climate change, will affect culture and social norms in ways we can scarcely begin to imagine.
Today, the line between the corporate capture of human experience, which gobbles up individual-data as “behavioral surplus” for prediction products that shape the space for consumer behavior, is beginning to blur to indecipherability, seeking to capture citizenship as well. It pursues the civic landscape through the same business models, marketing events, data evangelism, indeed through the same enterprise tech companies — leaving out, as always, the communities most impacted, to be acted upon by design.
“[This is] the
Datafication of Injustice…
in which the hunt for more and more data is a barrier to acting on what we already know”
– Ruha Benjamin, Race After Technology, p. 116
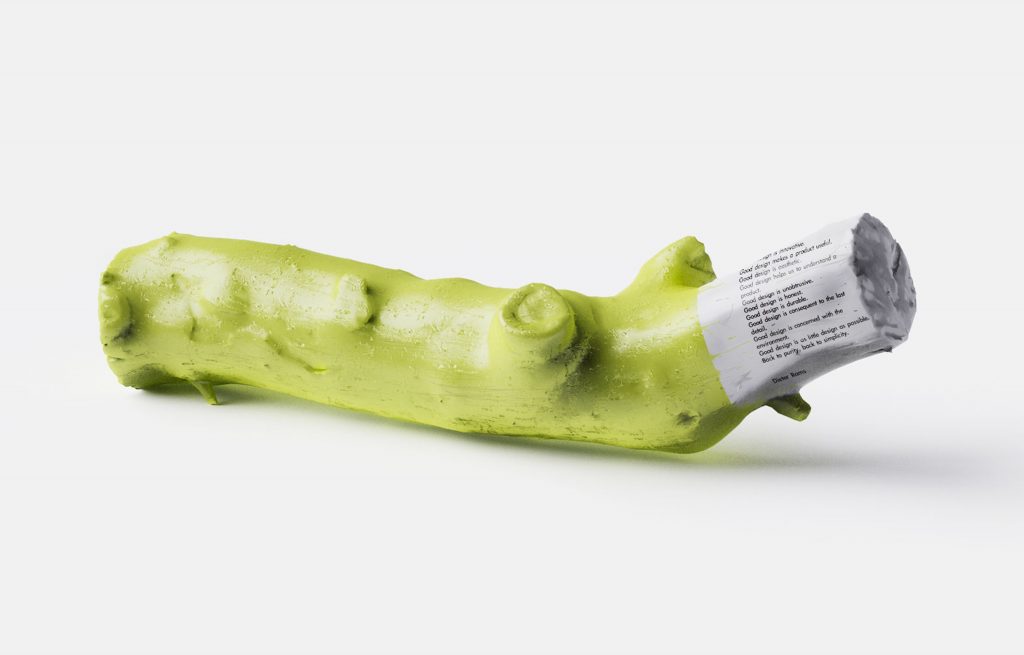
With our public systems of planning and civic management increasingly trained to frame complex systemic problems as individual in nature, we are effectively planning to blind our power structures to structural inequality. Focused on efficiency, we are doomed to deepen the grooves of inequity.
These are already systems designed in a paradigm of scientific management. Problems are viewed as linear and solvable through analytical, bureaucratic, and technocratic means. But the complex problems we face as a society (white supremacy, social and financial inequality, climate change, floating garbage islands, and many more) are interrelated, and exist, at least partially, due to the very systems designed to perform public problem-solving. The transformation necessary to better equip these systems to lift Americans up won’t happen through leveraging technology that is fundamentally about identifying and replicating — more efficiently and at scale — patterns of past service delivery.
The transformation needed is to equip locally administered systems with tools of collective action (the availability of descriptive data is only a small piece of this tool-kit) to work in concert with their community members and community-based organizations.
In most cases, communities already know what they need. The slow work of coalition-building and organizing, consensus building, and resourcing human capital just doesn’t often fit into the typically solutionist, scalability-obsessed, ROI focused, optimizing-for-efficiency, “evidence-based” funding hole carved out for “community innovation.”
And what is left for communities, unengaged on surveillance and algorithmic management — even where there has been a specific community engagement process — than to resist and refuse?
There is nothing inevitable about the application of predictive analytics to our public sector and social services. This is an elaborate delusion.
We desperately need civic infrastructure to reclaim planning for the future from its current technocratic neoliberal capitalist trajectory. We must extend new norms, laws, and protections for a world in which we must suddenly codify a right to sanctuary and autonomy in our own lives. This may even require a new language in order to move past what already feels like an outdated reliance on binaries like human|nonhuman and man|machine which in practice, only provide an intellectual cover for hierarchical oppression.
We need to recognize the colonial flavor of the data extraction suggested by technocratic approaches to community improvement. Institutions must accept responsibility for building the infrastructure to enable truly informed consent, without unduly burdening individuals. But it’s up to ordinary citizens to reclaim planning for the future, by telling different stories.
These stories might not have us at the center, or at least, not the “us” we think we understand. We might be more of a supporting character in a story that centers on the interconnectedness of all things as a central driver of purpose and experience. By failing to conceive of ourselves as the multivariate and interdependent ecosystems we are, we plan on a future that won’t include us if we can’t include the multitudes we contain and are contained by.